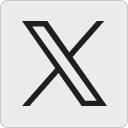


There is no question that AI-enabled technologies have the potential for significant positive impact for organizations overall, and for ITSM specifically. This recent TechTarget article highlights a number of positive business impacts resulting from the adoption of AI-enabled technologies, such as new capabilities and business model expansion, better quality, more innovation, and personalized customer services and experiences.
New and existing ITSM-related vendors are rushing into the space with solutions like AI-powered automation, conversational AI, intelligent chatbots, predictive analytics, and agentic AI (A web search on these terms will return numerous examples!).
And we’re only scratching the surface. New AI-enabled capabilities are on the horizon, such as:
- AI agents capable of executing discrete tasks independently based on personal preferences or providing customer service without requiring specific prompts.[i]
- AI-powered cybersecurity in the form of automated, near-constant backup procedures and AI tools for managing sensitive data to enhance data protection and resilience.[ii]
- Small Language Models (SLM) that aim to optimize models for existing use cases. SLMs can be trained on smaller, highly curated data sets to solve specific problems, rather than act on general queries (like Large Language Models).[iii]
But just because these rapidly-evolving technologies represent the “latest shiny new thing that really helps” (a tip of the cap to Paul Wilkinson) doesn’t mean that you should succumb to the fear of missing out by just “doing something”. In my experience, a new technology alone rarely (if ever) solves a business challenge. When it comes to technology investments, it’s better to make a good, informed decision, based upon the unique needs and challenges faced by your organization.
Yet, AI-related technologies can have and are having a significant positive impact on ITSM environments. Many organizations are already benefitting from the use of AI-enabled chatbots, automated ticket management, and service request automation.
The pressure to introduce AI-enabled capabilities to ITSM implementations is real. But which tools? What capabilities? How can one decide?
Five critical steps
Here are my five critical steps to making a good AI/ITSM decision.
- Define overarching goals for using AI within ITSM. It’s easy to become captivated by the latest products and features, especially in today’s AI/ITSM market frenzy. But chasing new products and features usually results in a short-sighted approach to technology adoption that will likely not meet longer term goals and needs. AI within ITSM should not be approached as a point solution; rather, AI should be considered within the broader perspective of ITSM. How will adding AI capabilities address current challenges? How will adding AI enable the organization to realize future ITSM objectives? Defining overarching goals for AI in ITSM – in business terms – ensures that broader perspective . Defining overarching goals also establishes the foundation for measuring AI/ITSM success.
- Conduct a SWOT analysis of the ITSM environment. Conducting a SWOT analysis identifies a company’s internal strengths and weaknesses, as well as external threats and opportunities. Understanding an organization’s ITSM SWOT identifies the critical factors that must be considered before developing an AI strategy. A SWOT is a good way to understand an organization’s readiness and ability to take on an AI initiative. Having the right stakeholders participate is critical to the success of a SWOT. Include stakeholders (especially non-IT colleagues) that have an interest in both ITSM and in AI capabilities and use. Include stakeholders that will freely share thoughts and ideas and have a pragmatic understanding of organizational issues and challenges.
- Develop the AI strategy. What is the approach for bringing in AI into your service management implementation? An effective AI strategy is not about finding places to “plug-in” an AI solution. It’s about understanding the organizational change, data, skills, budget, and infrastructure that will be needed for successfully utilizing AI technologies within the ITSM environment to help achieve the organization’s mission, vision, and goals. Use the results of the ITSM SWOT as an input to this strategy.
- Define evaluation criteria. The next step is to define the criteria by which potential AI solutions will be assessed. Defining this criteria up-front helps prevent falling victim to ‘shiny object syndrome’ and identify the solution that is best for your organization. As part of that criteria, consider the solutions alignment with the AI/ITSM strategy, costs (initial, ongoing, and cost effectiveness), the effectiveness of the solution to leverage issues identified in the SWOT, and how the solution enables the pursuit of potential future opportunities.
- Develop and present the business case. Gaining and maintaining the commitment of senior management is critical for success. When a potential solution is found, develop and present the business case for that solution. Discuss the technical and cultural challenges that come with AI adoption. Discuss the opportunities that AI with ITSM will provide. Discuss how a solution will address SWOT and align with the AI strategy. Discuss the benefits of implementing the solution , how risks will be optimized, and how success will be measured. Discuss the consequences of doing nothing. Most importantly, ask for management commitment.
Cautions
Before moving forward with introducing AI within an ITSM environment, here are some cautions of which to be aware.
- Good AI will not fix bad ITSM. The adoption of AI technologies can enable and enhance ITSM capabilities. However, AI is not a “magic wand” that solves issues like poor process design, inadequate service management governance, and ineffective measurement and reporting.
- Don’t overlook data quality and governance. Many organizations have data quality and data governance challenges. AI needs data – lots of it – and that data must be accurate, reliable, and trustworthy. Data quality and governance is not just a challenge for ITSM, it is an organizational problem.
- Is there an ITSM strategy? Many organizations are not achieving the full potential of ITSM adoption. Rather than applying ITSM holistically, many implementations have only focused ITSM implementation on IT operational issues, and not on how ITSM enables business outcomes. Without an overarching ITSM strategy, AI investments risk becoming short-sighted and expensive point solutions that do not address business needs.
Augmenting the ITSM environment with the right AI capabilities can be a huge benefit for the organization, ITSM, and the employees of an organization. But introducing AI within ITSM is not a decision to be taken lightly. Taking a systemic approach to identifying, justifying, and selecting solutions sets the right expectations with stakeholders and helps ensure successful introduction of ITSM with AI.
[i] https://www.uc.edu/news/articles/2025/01/innovation-experts-predict-top-tech-trends-for-2025.html , Retrieved January 2024.
[ii] Ibid.
[iii] https://www2.deloitte.com/content/dam/insights/articles/us187540_tech-trends-2025/DI_Tech-trends-2025.pdf , Retrieved January 2024.
Share
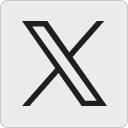

