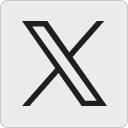
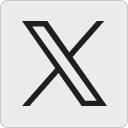
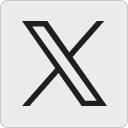






















There’s no question that introducing AI capabilities can have a dramatic impact on IT Service Management (ITSM). Done well, AI adoption will free up ITSM professionals to do the work for which humans are uniquely qualified, like critical thinking, contextual understanding, and creative problem-solving. Furthermore, AI will enable organizations to realize many of the theoretical benefits of ITSM. For example, the use of AI and machine learning can leverage comprehensive in-depth data, not just a small recent sampling, for cause analysis, problem detection, and impact determination of problems. Another example is the use of AI can increase the data of the IT environment and automate the remediation of incidents.
But AI is not a “magic wand” for ITSM.
Before introducing AI capabilities into ITSM, organizations must first consider these three AI truths.
Truth #1 – AI needs good data
For the use of AI to be effective, it needs data. Lots of data. But, if that data is inaccurate, lacks integrity, or is not trustworthy, then the use of AI will only produce inaccurate or poor results.
Data quality is an issue that many organizations will have to tackle before realizing the complete benefits of introducing AI to their ITSM implementations. These means that organizations will have to step up their technology and data governance posture. According to this recent Privacera article, a fundamental principle of data governance is having a high-quality, trusted data source. Having trusted data sources enables capabilities like ITSM to make accurate and reliable decisions regarding service management issues. But if the data sources used by ITSM tools contain data that is unregulated, the ability to automate responses is significantly hindered.
Truth #2 – AI doesn’t mean process design goes away
The need for effective ITSM processes and procedures doesn’t go away with AI adoption. Machine learning can be used to detect data patterns to understand what was done to resolve an issue. But what machine learning doesn’t do is determine if what is being done is the best approach. Machine learning doesn’t consider organizational goals and objectives with the adoption of ITSM. Machine learning cannot determine what processes are missing or need improvement to gain needed effectiveness and efficiency with ITSM.
Truth #3 – AI doesn’t replace knowledge
“Reducing cost”, often in the form of headcount reductions, is frequently used as the justification for AI investment, as the use of AI will enable ITSM activities to be automated. And it’s true – many of the ITSM activities currently performed by humans can and should be replaced with AI-enabled capabilities, such as the automated fulfilment of service requests, automated response to incidents, and problem data analysis. But one of the hidden costs of using AI to justify headcount reductions is the form of knowledge loss – the knowledge inside people’s heads walks out the door when their positions are eliminated. And this is the knowledge that is critical for training the chatbots, developing the LLMs needed, and to the continual improvement of AI and ITSM.
While AI can provide the “how” for “what” needs to be done, it cannot answer the “why” it needs to be done.
Good Governance facilitates AI-enabled ITSM
Without governance, AI can do some serious damage, not just with ITSM, but to the organization. As the role of IT organizations shifts from being data owners (often by default) to being data custodians, having well defined and enforced policies regarding data governance is critical. This means that the frequently found approach to governance consisting of an IT track and a corporate track is becoming untenable. As organizational processes and workflows become increasingly automated, enabled by AI capabilities, governance must become cross-functional[i] , with sales, marketing, HR, IT, and other organizational functions all involved. Organizations must consider and address data-related issues such as compliance with data privacy laws, ethical data use, data security, data management, and more.
An effective approach to governance enables organizations to define their digital strategy[ii] to maximize the business benefits of data assets and technology-focused initiatives. A digital strategy produces a blueprint for building the next version of the business, creating a bigger, broader picture of available options and down-line benefits.[iii] Creating a successful digital strategy requires an organization to carefully evaluate its systems and processes, including ITSM processes. And as ITSM processes are re-imagined for use across the enterprise in support of organizational value streams, effective governance becomes essential.
Getting ready for AI-enabled ITSM
What are some of the first steps organizations should take to get ready for AI-enabled ITSM?
- Formalize continual improvement. One of the most important practices of an effective ITSM implementation is continual improvement. As organizations are continually evolving and changing, continual improvement ensures that ITSM practices evolve right alongside those business changes. And just like service management, AI adoption is not an “implement and forget”; in fact, AI will absolutely fail without formal continual improvement.
- Answer the “why”. To say that there is so much hype around the use of AI within ITSM would be an understatement. Before jumping into AI, first develop and gain approval of the business case for using AI within ITSM. How will success be determined and measured? What opportunities for innovation will emerge by relieving people from performing those tedious and monotonous tasks associated with the current ITSM environment? What returns will the organization realize from the use of AI within ITSM? What new business or IT opportunities may be available because of the use of AI within ITSM? A good business case establishes good expectations for the organization regarding AI and ITSM.
- Begin thinking about how AI can be leveraged by ITSM process designs. As discussed in this recent HBR.org article, AI will bring new capabilities to business (and ITSM) processes. With these new capabilities, organizations will need to rethink what tasks are needed, who will do those tasks, and the frequency that those tasks will be performed. The use of AI will enable organizations to rethink their ITSM processes from an end-to-end perspective, considering what tasks should be performed by people and what tasks should be performed by machines.
The concept of augmenting ITSM with AI is a “no-brainer”. However, success with AI in an ITSM environment requires a lot of up-front thought, good process design, solid business justification, and considering these three AI truths.
[i] https://2021.ai/ai-governance-impact-on-business-functions
[ii] https://www.techtarget.com/searchcio/definition/digital-strategy
[iii] Ibid.
Share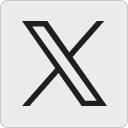
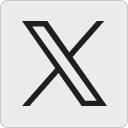
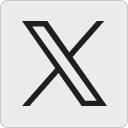





















